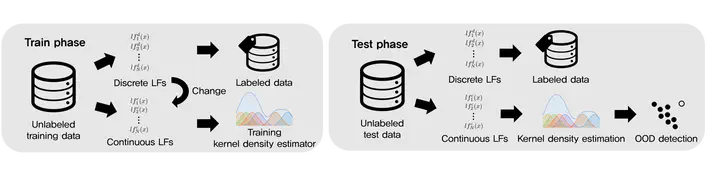
Project summary
Detecting domain shift and updating ML pipeline appropriately is crucial in real-world ML systems. We argue that using labeling function outputs rather than downstream model outputs is a more effective method for detecting domain shift. We propose a lightweight and simple framework that converts discrete labeling functions to continuous functions and applies density estimation method to detect outof-distribution samples in test time. We analyze the performance of our proposed approach on three real-world datasets on binary sentiment analysis task, and show that our approach is effective at detecting domain shift.
Libraries and frameworks
- PyTorch